(in a long line of LiWC-like lexicons) Chenhao Tan's list of hedging phrases, such as "I suspect", "raising the possibility": [README] [list itself] Expand |
---|
title | References and applications |
---|
| Chenhao Tan and Lillian Lee, "Talk it up or play it down? (Un)expected correlations between (de-)emphasis and recurrence of discussion points in consequential U.S. economic policy meetings", Text As Data 2016 Abstract: In meetings where important decisions get made, what items receive more attention may influence the outcome. We examine how different types of rhetorical (de-)emphasis — including hedges, superlatives, and contrastive conjunctions — correlate with what gets revisited later, controlling for item frequency and speaker. Our data consists of transcripts of recurring meetings of the Federal Reserve’s Open Market Committee (FOMC), where important aspects of U.S. monetary policy are decided on. Surprisingly, we find that words appearing in the context of hedging, which is usually considered a way to express uncertainty, are more likely to be repeated in subsequent meetings, while strong emphasis indicated by superlatives has a slightly negative effect on word recurrence in subsequent meetings. We also observe interesting patterns in how these effects vary depending on social factors such as status and gender of the speaker. For instance, the positive effects of hedging are more pronounced for female speakers than for male speakers. Chenhao Tan, Vlad Niculae, Cristian Danescu-Niculescu-Mizil, Lillian Lee. "Winning arguments: Interaction dynamics and persuasion strategies in good-faith online discussions." Proc. of WWW 2016 |
- language models, which assign probabilities P(x) to words, sentences or text units.
These are great for similarity, distinctiveness, visualization.
Monroe et al's "Fightin words": what makes two "languages" different? Slides and handout from Cristian Danescu-Niculescu-Mizil and my class "NLP and social interaction" : [ pptx ] [ pdf ] [handout] Expand |
---|
title | (Test-audience-familiarity image) |
---|
| 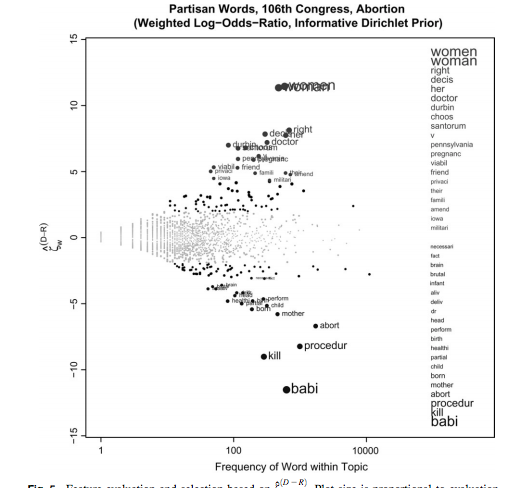
|
Expand |
---|
title | Applications of the method |
---|
| Jurafsky, Dan, Victor Chahuneau, Bryan R. Routledge, Noah A. Smith. 2014. Narrative framing of consumer sentiment in online restaurant reviews. First Monday 19(4). Mark Liberman on Language Log. The most Kasichoid, Cruzian, Trumpish, and Rubiositous words , 2016. The most Trumpish (and Bushish) words , 2015. Obama's favored (and disfavored) SOTU words , 2014. Draft words (descriptions of white vs black NFL prospects), 2014. Male and female word usage , 2014. |
Expand |
---|
| Monroe, Burt L., Michael P. Colaresi, and Kevin M. Quinn. 2008. Fightin' words: Lexical feature selection and evaluation for identifying the content of political conflict . Political Analysis 16(4): 372-403. [alternate link] Abstract: Entries in the burgeoning “text-as-data” movement are often accompanied by lists or visualizations of how word (or other lexical feature) usage differs across some pair or set of documents. These are intended either to establish some target semantic concept (like the content of partisan frames) to estimate word-specific measures that feed forward into another analysis (like locating parties in ideological space) or both. We discuss a variety of techniques for selecting words that capture partisan, or other, differences in political speech and for evaluating the relative importance of those words. We introduce and emphasize several new approaches based on Bayesian shrinkage and regularization. We illustrate the relative utility of these approaches with analyses of partisan, gender, and distributive speech in the U.S. Senate.
The method is also described in Section 19.5.1, "Log odds ratio informative Dirichlet prior" of the 3rd edition of Jurafsky and Martin, Speech and Language Processing. Slides adapted from slides 85-94 of Cristian Danescu-Niculescu-Mizil and Lillian Lee, Natural language processing for computational social science, Invited tutorial at NIPS 2016 [alternate link: tutorial announcement, slides] for lecture 16 of the class NLP and Social Interaction, Fall 2017. Code - Hessel, Jack: FightingWords.
- Lim, Kenneth: fightin-words 1.0.4. Compliant with sci-kit learn and distributed by PyPI; borrows (with acknowledgment) from Jack's version.
- Marzagão, Thiago: mcq.py
Visualizers |
- Similarity measured on the most frequent words ("stop words") only vs. on the content words
How similar are two language models? The standard measure is the cross-entropy: - Σ p( x) log( q(x)) ; a variant is the KL divergence, Σ p(x) log( p(x) / q(x)) = the cross entropy of p(x) and q(x) minus the entropy of p(x) Similarity of each of A or B to a baseline of "regular" or "null hypothesis" language.
Distributional similarity (word embeddings are the modern version) Here's a figure from 1997 about ideas from the early 90's: 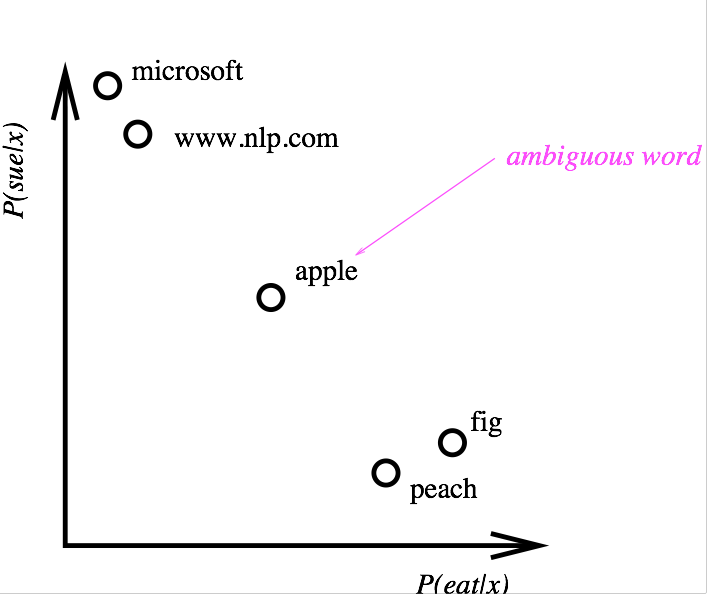 For references, see the word embeddings section later in this document Expand |
---|
title | Should we measure distance using regular ol' vector differences? Pictures from an intuition |
---|
| 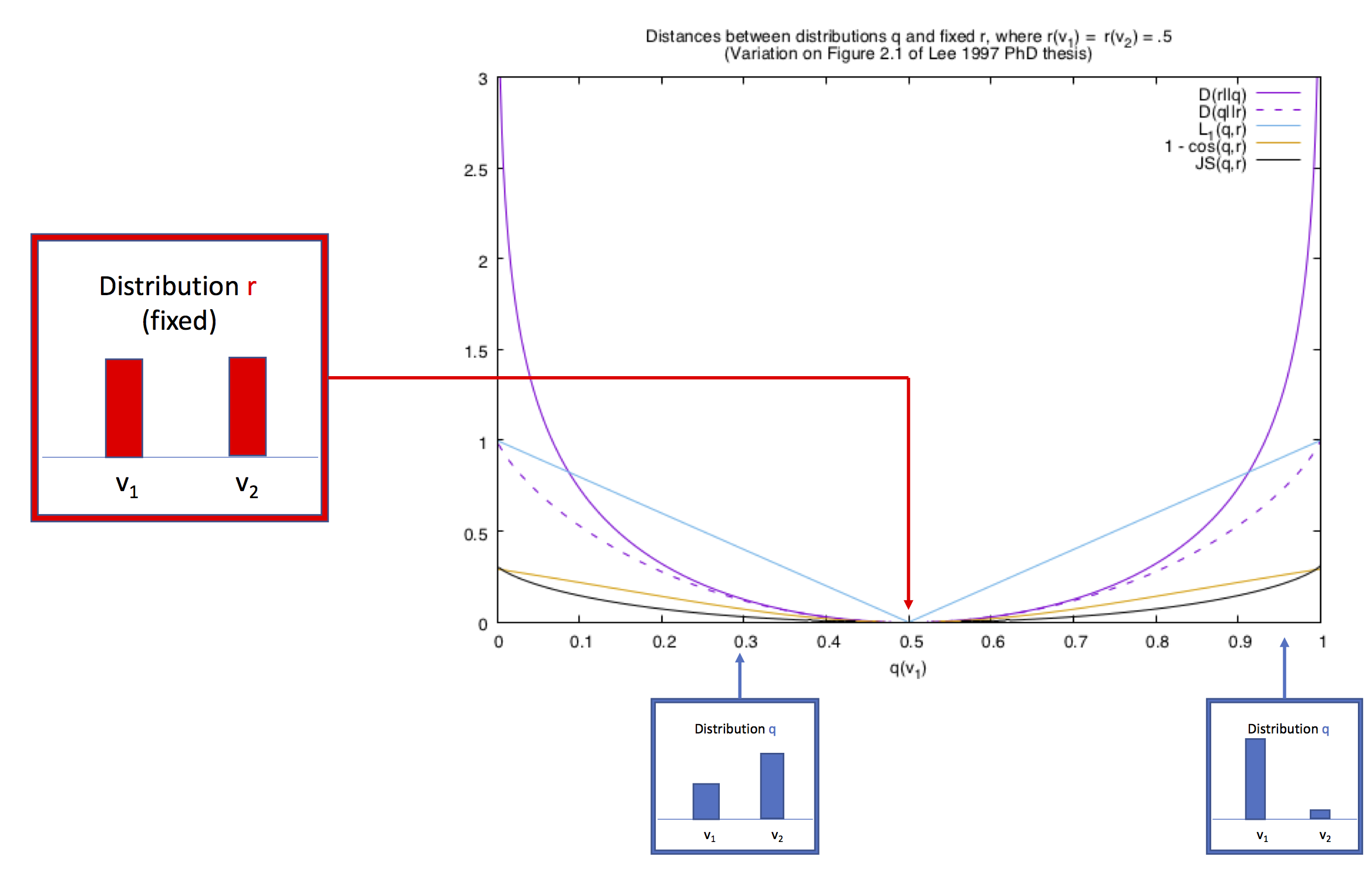
|
Some others I don't expect to have time to discuss Expand |
---|
title | Show us those mystery features! |
---|
| Type/token ratio |
|